Do you see the pattern?
How does pattern recognition make sure that the bottle bank pays out the correct refund, that the farmer sprays only the weeds in his fields, and that Hydro Aluminium saves money?
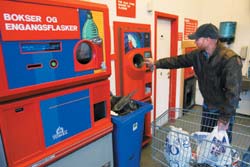
In the new Tomra 600 reverse vending machines, video cameras are used to recognize the shapes of different types of bottle, allowing them to be guided correctly through the machine and giving the customer the correct deposit return.
Photo: Rune Petter Ness
A tractor rumbles slowly across the yellow-green field. A down-facing screen mounted on its front scans the ground. It contains a video-camera and lights, so that good pictures can be taken no matter what the weather is like.
The aim is to track down the enemy – weeds. Instead of spraying weed-killer all over the field, the farmer wants to spray only weed-infested areas. Information gathered by the camera is transmitted to the spraying unit mounted on the back of the tractor, which releases a pulse of herbicide only when necessary – and helps to reduce the consumption of chemical by the agriculture industry.
But how can this system distinguish weeds from all the other types of plants on the ground? And how are different types of weeds “sniffed up”?
“We identify properties and information in these images that can help us to discriminate among different objects and recognize them again”, says SINTEF research scientist Helene Schulerud. “Corn, for example, is long and slim in shape, while the leaves of most weeds are rounder”.
The scientists are developing a computer program that starts by distinguishing the leaves from the soil itself. Then they estimate the shapes of the corn and the various weeds and create rules that classify these and differentiate them from one another. When the video-camera sends images to the computer they can be analysed in real time, the individual leaf shapes are recognized, and the degree of weed infestation and the amount of herbicide required can be determined.
Separate field of research
The project is one example of the invaluable help that pattern recognition can provide. Pattern recognition is a distinct, widely ranging and growing field of research. The method is used to tackle problems in which the quantity of data is too large to handle, or where the questions involved are diffuse and difficult to get to grips with.
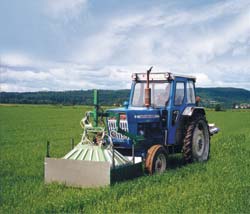
A weed detector is mounted at the front of the tractor in the form of a screen with its opening facing down. The camera sends pictures to a computer which analyses the shapes of the leaves.
Photo: Hein Stavlund, DAT AS
Many scientists are working in this area. Besides SINTEF, the Norwegian Computing Centre, several university research groups and some private companies are active.
Pattern recognition processing can be carried out on all sorts of data, but it is particularly relevant for image processing. Greater processing power and drops in prices have turned digital cameras into attractive sensors and image analysis into an active field of research. In SINTEF’s Dept. of Optical Measurement Systems and Data Analysis, 15 scientists are working on various aspects of optics, light and image analysis, and pattern recognition is a central aspect of their work.
“Our strength lies in our ability to look at the measurement problem as a whole”, says chief scientist Erik Wold. “For every individual task, we have to work out a measurement method and lighting set-up that gives us the best images. Then we analyze the images and develop the algorithms we need to solve the task”.
Image, description, analysis
The first step taken by scientists is to get hold of images of the object that are as full of information as possible. Correct lighting is essential to give the computer a good point of departure. Depending on what the client needs to measure, the image will not be a normal picture, but instead may emphasize temperature differences, shapes or characteristics of the material. Such images can be captured by a video camera, a web camera or through a microscope, or they may be synthetic, i.e. generated from other types of measurement data.
The next stage is the description. This is a matter of extracting information that distinguishes the objects in the image from one another, or the object that we are looking for from the other figures in the picture.
Helene Schulerud explains: “If you want to train a computer to recognize an image of the letter B, for example, you need to describe the letter in terms of a set of characteristic features such as the number of vertical and horizontal lines and the area that it occupies. In the same way, we can distinguish weeds from other plants, or sort out the movements of a
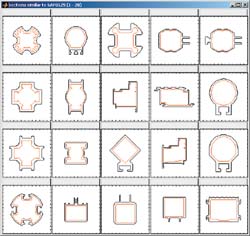
Cross-sections of extruded aluminium profiles for Hydro Aluminium. Pattern recognition
techniques are used to find similarities between earlier produced aluminium profiles and new ones. Based on the similarities, the process parameters for the new aluminium profile are found.
thief from natural movements in an area under surveilolance”.
“In many cases it is a fairly simple matter to get the system to recognize a target object in certain images”, says Wold, “but being able to pick out the same object in all the new pictures that are taken under different conditions is a more difficult task that requires a robust system”.
Bottles and aluminium profiles
Automatic pattern recognition is by no means a new field. For many years, the Norwegian company Tomra Systems has been using technology developed by SINTEF in its reverse vending (bottle return) machines.
The new generation of machines introduced in 1997 use video cameras to recognize individual bottle shapes. Robust recognition means that the bottles are sorted correctly and that the customer is given the correct refund for each individual bottle. The combination of advanced analytical techniques and inexpensive technology has helped to give Tomra its dominant position in today’s bottle return market.
Hydro Aluminium is another user of pattern recognition. Metal is extruded into aluminium profiles by forcing a bolt of molten aluminium through a nozzle. The correct temperature and pressure are essential for successful production of the final profile. The process operators try out various process parameters (measurement values) in order to gain experience. A good result is eventually obtained and the correct settings are noted. Next time, however, new profiles have to be produced. Will they have to begin from the beginning again, or can the «old» profiles be reused?
“Hydro has an archive of tens of thousands of profiles in production, so there is no point in just looking for similar profiles with the naked eye”, says Schulerud. “Our research tries to describe which features make profiles ‘look the same’, and thus have similar parameters. These might be a matter of thickness, the number of fins that stick out, or whatever.
Pattern recognition helps us to automatically identify the old profiles that are most like the new ones we wish to produce. The operator has a good point of departure from which to estimate his process parameters more quickly. Experience gained in producing previous profiles can also give him useful tips as to how the tools should be formed. As a company,
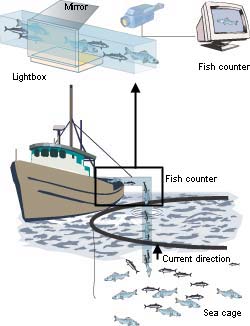
A video camera, lighting system, mirror and computer are used to photograph a fish from various angles as it passes through a transparent section of the pipe in which it is pumped from the sea-cage to the boat. The images allow the volume and weight of the fish to be estimated. The fish slaughterhouse can upgrade the quality of its work, and the fish farmer knows just how much he has delivered.
Illustration: Jan H. Johansen, SINTEF Media
Hydro avoids a great deal of trial and error and improves its productivity”.
FACTS
Pattern recognition is a field of research that deals with the design of systems for recognizing patterns in data. The first step is to create a model, often with the aid of known data. The model is then used to classify new, unknown data.
Pattern recognition can be used to:
- recognize faces in a crowd
- classify and identify fingerprints
- distinguish normal cells from cancerous cells
- identify faulty items in a production line
- recognize text in a picture
- recognize people’s shopping habits in stores (compute-
- rized shops)
- identify potential pockets of oil and gas in seismic data
- expose financial swindlers
- expose terrorists!
Pattern recognition often replaces manual inspections and can be incorporated in mechanical vision systems for industrial inspection tasks. The advantages of pattern recognition are that it is rapid, the same feature is measured objectively time after time, and it never gets tired.
Video surveillance
FACTS
Pattern recognition is a field of research that deals with the design of systems for recognizing patterns in data. The first step is to create a model, often with the aid of known data. The model is then used to classify new, unknown data.
Pattern recognition can be used to:
- recognize faces in a crowd
- classify and identify fingerprints
- distinguish normal cells from cancerous cells
- identify faulty items in a production line
- recognize text in a picture
- recognize people’s shopping habits in stores (compute-
- rized shops)
- identify potential pockets of oil and gas in seismic data
- expose financial swindlers
- expose terrorists!
Pattern recognition often replaces manual inspections and can be incorporated in mechanical vision systems for industrial inspection tasks. The advantages of pattern recognition are that it is rapid, the same feature is measured objectively time after time, and it never gets tired.
Every type of security system that relies on video surveillance is based on the same principle: video cameras are connected to a computer, and the images recorded are analysed in order to determine whether something unusual is taking place in the area under surveillance. Or in other words, whether the normal pattern is being broken.
If every single change in the image produced a response, the alarm would sound continuously. For this reason, all «unalarming» situations need to be suppressed or ignored by the system.
Since 1992, SINTEF has been collaborating with Videoweb 1 (formerly, Detec) in the development of an intelligent automatic video surveillance system. The project has emphasized the recognition of occurrences that modify the image but are not particularly suspicious, such as changes in lighting, or trees that move in the wind. This is done by means of pattern recognition. The system concentrates on features of the image that do not alter during such changes. This avoids stressing the guard, who is thus able to monitor several cameras at the same time.
Text: ÅSE DRAGLAND